In anticipation of the forthcoming GrowthBook 3.0 release, we’re making several changes to how our Bayesian engine works to enable specifying your own priors, bring variance reduction via CUPED to the Bayesian engine, and improve estimation in small sample sizes.
What does this update mean for your organization? In some cases, you may notice a slight shift in the results for existing experiments. However, the magnitude of these shifts is minimal, only applies in certain cases, and serves to enhance the power of our analysis engine (jump to summary of changes).
This post will give a high level overview of what’s changing, why we changed it, and how it will affect results. You can also check out the content of this blog in video form using the video below:
The new Bayesian model
In Bayesian inference, we leverage a prior distribution containing information about the range of likely effects for an experiment. We combine this prior distribution with the data to produce a posterior that provides our statistics of interest — percent change, chance to win, and the credible interval.
The key difference in our new Bayesian engine is that priors are specified directly on treatment effects (e.g. percent lift) rather than on variation averages and using those to calculate experiment effects. With this update, you only need to think about how treatment will affect metrics instead of providing prior information for both the control variation and the treatment variation. This simplifies our Bayesian engine and the work involved for you.
This change is largely conceptual for many customers. If you’re interested in the details, you can read about the new model here and the old model in the now outdated white paper.
3 key benefits of the new model
1) Specify your own priors
The previous model required at least 4 separate values to be specified for every metric in order to set custom priors. This involved more effort to come up with reasonable values.
Now, you set a single mean and standard deviation for your prior for the relative effects of experiments on your metric. For example, a prior mean of 0 and a standard deviation of 0.3 (our defaults if you turn on priors) captures the prior knowledge that the average lift is 0% and that ~95% of all lifts are between -60% and 60%, in line with our existing customer experiments.
The default is not to use prior information at all, but you are able to turn it on and customize it at the organization level, the metric level, and at the experiment-metric level.
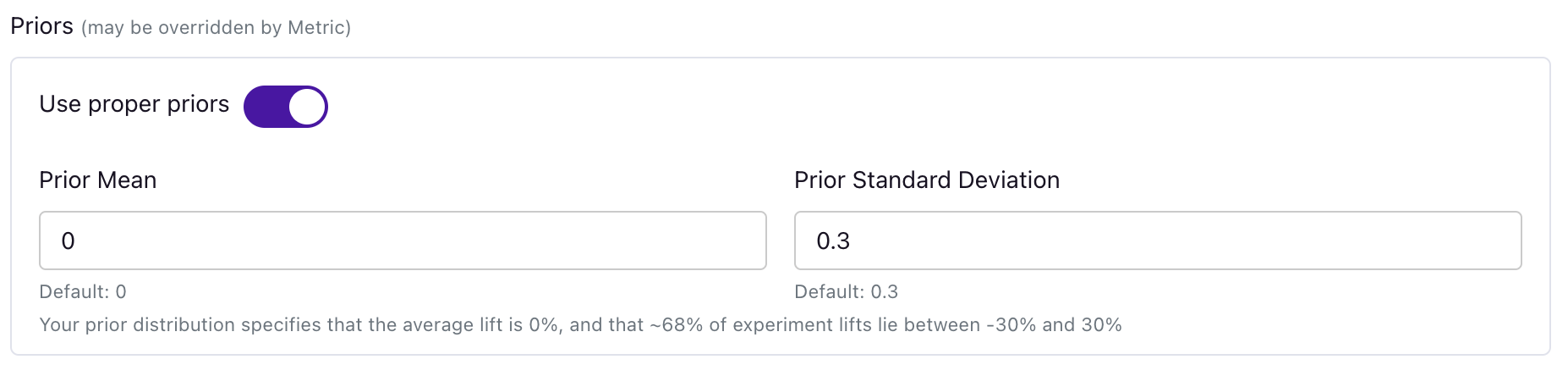
2) CUPED is now available in the Bayesian engine
By modeling relative lifts directly, the new model unlocks CUPED in the GrowthBook Bayesian engine for all Pro and Enterprise customers. CUPED uses pre-experiment data to reduce variance and speed up experimentation time and can be used with either statistics engine in GrowthBook. You can read a case study about how powerful CUPED is here and you can read our documentation on CUPED here.
3) Fewer missing results with small sample sizes
Our old model worked only when the model was reasonably certain that the average in the control variation was greater than zero. When the control mean was near zero, the log approximation we previously relied on could return no chance to win or credible intervals (CI). You might have seen something that looked like the following:

The 50% is a placeholder as we could not compute the inference and the CI is missing. This is somewhat frustrating, given that there’s almost 3k users in this experiment! In the new model, we do not have the same constraints and we can instead return the following, more reasonable results:

How does it affect existing estimates?
Any new experiment analyses, whether that be a new experiment or a results refresh for an old experiment, will use the new model. If the last run before refreshing results used the old model, results could shift slightly even if you do not use the new prior settings.
- For proportion/binomial/conversion metrics, the % change could shift, along with chance to win and the CI. However, these shifts should be minimal (< 3 percentage points) in most cases, especially if the variations are equal size.
- For revenue/duration/count/mean metrics, the % change should not shift at all, but the chance to win and the CI could change slightly (again up to around 3 percentage points except in some edge cases).
For example, here’s a typical proportion metric and what it looks like before and after the change.
Before:

After:

The changes for revenue metrics (and other count or mean metrics) should be even less pronounced on average.
Were the old results incorrect?
No.
The results from the old Bayesian model were not less accurate or incorrect. In general, Bayesian models can take many forms, each with their own pros and cons. The old model was tuned to specify priors for each variation separately, which made it highly customizable but more tedious to set up. The new model makes setting priors easier and improves our ability to compute key inferential statistics in small sample sizes.
The new model moves the Bayesian machinery to focus on experiment lifts; this allows us to leverage approaches like the Delta method to compute the variance for relative lifts as well as CUPED to make the new model more tractable in certain edge cases and more powerful for most users.
Feel free to read more about the statistics we use in our documentation here or reach out in our community slack here.